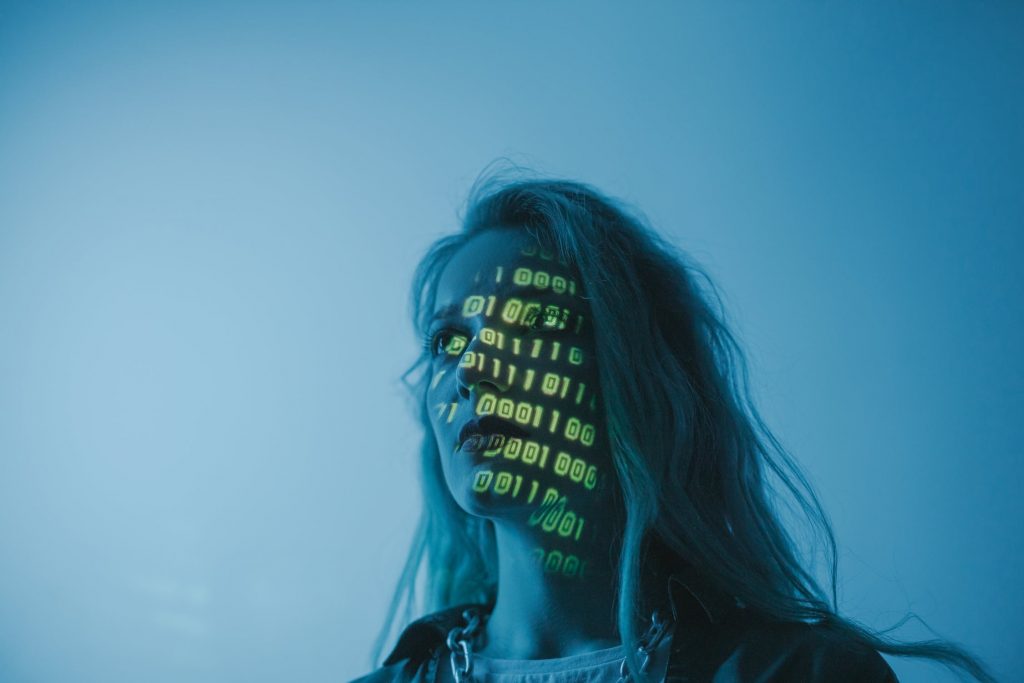
GUEST POST from Pete Foley
For those of us working in the innovation and change field, it is hard to overstate the value and importance of AI. It opens doors, that were, for me at least, barely imaginable 10 years ago. And for someone who views analogy, crossing expertise boundaries, and the reapplication of ideas across domains as central to innovation, it’s hard to imagine a more useful tool.
But it is still a tool. And as with any tool, leaning it’s limitations, and how to use it skillfully is key. I make the analogy to an automobile. We don’t need to know everything about how it works, and we certainly don’t need to understand how to build it. But we do need to know what it can, and cannot do. We also need to learn how to drive it, and the better our driving skills, the more we get out of it.
AI, the Idiot Savant? An issue with current AI is that it is both intelligent and stupid at the same time (see Yejin Chois excellent TED talk that is attached). It has phenomenal ‘data intelligence’, but can also fail on even simple logic puzzles. Part of the problem is that AI lacks ‘common sense’ or the implicit framework that filters a great deal of human decision making and behavior. Chois calls this the ‘dark matter’ common sense of decision-making. I think of it as the framework of knowledge, morality, biases and common sense that we accumulate over time, and that is foundational to the unconscious ‘System 1’ elements that influence many, if not most of our decisions. But whatever we call it, it’s an important, but sometimes invisible and unintuitive part of human information processing that is can be missing from AI output.
Of course, AI is far from being unique in having limitations in the quality of its output. Any information source we use is subject to errors. We all know not to believe everything we read on the internet. That makes Google searches useful, but also potentially flawed. Even consulting with human experts has pitfalls. Not all experts agree, and even to most eminent expert can be subject to biases, or just good old fashioned human error. But most of us have learned to be appropriately skeptical of these sources of information. We routinely cross-reference, challenge data, seek second opinions and do not simply ‘parrot’ the data they provide.
But increasingly with AI, I’ve seen a tendency to treat its output with perhaps too much respect. The reasons for this are multi-faceted, but very human. Part of it may be the potential for generative AI to provide answers in an apparently definitive form. Part may simply be awe of its capabilities, and to confuse breadth of knowledge with accuracy. Another element is the ability it gives us to quickly penetrate areas where we may have little domain knowledge or background. As I’ve already mentioned, this is fantastic for those of us who value exploring new domains and analogies. But it comes with inherent challenges, as the further we step away from our own expertise, the easier it is for us to miss even basic mistakes.
As for AI’s limitations, Chois provides some sobering examples. It can pass a bar exam, but can fail abysmally on even simple logic problems. For example, it suggests building a bridge over broken glass and nails is likely to cause punctures! It has even suggested increasing the efficiency of paperclip manufacture by using humans as raw materials. Of course, these negative examples are somewhat cherry picked to make a point, but they do show how poor some AI answers can be, and how they can be low in common sense. Of course, when the errors are this obvious, we should automatically filter them out with our own common sense. But the challenge comes when we are dealing in areas where we have little experience, and AI delivers superficially plausible but flawed answers.
Why is this a weak spot for AI? At the root of this is that implicit knowledge is rarely articulated in the data AI scrapes. For example, a recipe will often say ‘remove the pot from the heat’, but rarely says ‘remove the pot from heat and don’t stick your fingers in the flames’. We’re supposed to know that already. Because it is ‘obvious’, and processed quickly, unconsciously and often automatically by our brains, it is rarely explicitly articulated. AI, however, cannot learn what is not said. And so because we don’t tend to state the obvious, it can make it challenging for an AI to learn it. It learns to take the pot off of the heat, but not the more obvious insight, which is to avoid getting burned when we do so.
This is obviously a known problem, and several strategies are employed to help address it. These include manually adding crafted examples and direct human input into AI’s training. But this level of human curation creates other potential risks. The minute humans start deciding what content should and should not be incorporated, or highlighted into AI training, the risk of transferring specific human biases to that AI increase. It also creates the potential for competing AI’s with different ‘viewpoints’, depending upon differences in both human input and the choices around what data-sets are scraped. There is a ‘nature’ component to the development of AI capability, but also a nurture influence. This is of course analogous the influence that parents, teachers and peers have on the values and biases of children as they develop their own frameworks.
But most humans are exposed to at least some diversity in the influences that shape their decision frameworks. Parents, peers and teachers provide generational variety, and the gradual and layered process that builds the human implicit decision framework help us to evolve a supporting network of contextual insight. It’s obvious imperfect, and the current culture wars are testament to some profound differences in end result. But to a large extent, we evolve similar, if not identical common sense frameworks. With AI, the narrower group contributing to curated ‘education’ increases the risk of both intentional and unintentional bias, and of ‘divergent intelligence’.
What Can We do? The most important thing is to be skeptical about AI output. Just because it sounds plausible, don’t assume it is. Just as we’d not take the first answer on a Google search as absolute truth, don’t do the same with AI. Ask it for references, and check them (early iterations were known to make up plausible looking but nonsense references). And of course, the more important the output is to us, the more important it is to check it. As I said at the beginning, it can be tempting to take verbatim output from AI, especially if it sounds plausible, or fits our theory or worldview. But always challenge the illusion of omnipotence that AI creates. It’s probably correct, but especially if its providing an important or surprising insight, double check it.
The Sci-Fi Monster! The concept of a childish super intelligence has been explored by more than one Science Fiction writer. But in many ways that is what we are dealing with in the case of AI. It’s informational ‘IQ’ is greater than the contextual or common sense ‘IQ’ , making it a different type of intelligence to those we are used to. And because so much of the human input side is proprietary and complex, it’s difficult to determine whether bias or misinformation is included in its output, and if so, how much? I’m sure these are solvable challenges. But some bias is probably unavoidable the moment any human intervention or selection invades choice of training materials or their interpretation. And as we see an increase in copyright law suits and settlements associated with AI, it becomes increasingly plausible that narrowing of sources will result in different AI’s with different ‘experiences’, and hence potentially different answers to questions.
AI is an incredible gift, but like the three wishes in Aladdin’s lamp, use it wisely and carefully. A little bit of skepticism, and some human validation is a good idea. Something that can pass the bar, but that lacks common sense is powerful, it could even get elected, but don’t automatically trust everything it says!
Image credits: Pexels
Sign up here to join 17,000+ leaders getting Human-Centered Change & Innovation Weekly delivered to their inbox every week.
Pingback: Top 10 Human-Centered Change & Innovation Articles of January 2024 | Human-Centered Change and Innovation